Analyzing and Interpreting Results
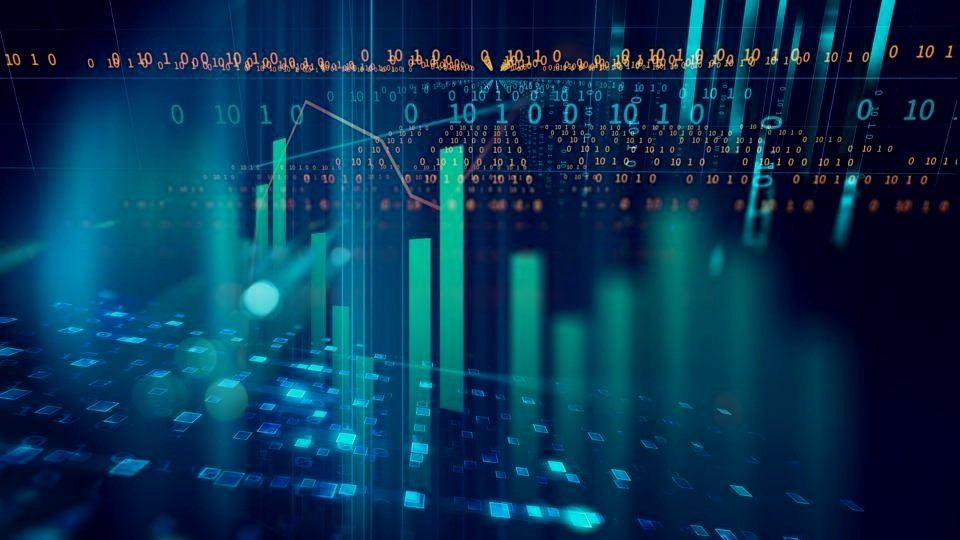
Analyze & Interpret Results:
General advice: analyzing and interpreting your data is likely the 2nd most important aspect of research. Data collection is the 1st most important and involves things like experimental design and controls and performing the actual experiments, but then to translate raw findings into something intelligible is the actual part of science where you get your findings and ultimately draw conclusions. Something important to understand is that you can interpret the same results to draw differing conclusions. So staying open minded as well as physically spending a lot of time with your own data is important. Your own intuition can surprise you and offer insights that might not be obvious if you just glance at the results. Typically you will spend lots of the time graphing or visualizing your data into different forms or formats, so spending time with your data comes natural. But just in case you are ever in a rush, it pays off to go back and look at your raw data to make sure there are not any strange errors and to sub consciously scan for things that might not be obvious at first.
Data analysis
- Raw data: This is what you’ll find in your lab notebooks or excel sheets or images. It’s the thing that you have collected and have not analyzed yet. Depending on what you do, this may not be the output of a particular experimental device. For example, in RNA sequencing, the counts table that contains your quantification of transcripts is often an output from the sequencing core facility. But in reality, your raw data is the fastq file that contains the actual reads, which is the most raw or unanalyzed version of your data.
o Organization: it is vital that you organize your data in a manner that will be easily used by someone other than yourself. A good rule of thumb is to try to keep your data and lab notebook in such a way that if you were to die, someone could easily continue your work without needing to talk to you.
§ Dates; I suggest naming files with the format ‘yyyy.mm.dd’ so that when you sort that folder of files, you can go back and search through chronological order.
§ Labels; put as much information on the label as possible. The date it was made/collected, the info on the sample, sample number, and then right in your lab notebook where it is stored.
§ Storage; all data must be backed up according to the NIH. If you don’t work with nih money, than you should still back up your data onto some sort of lab server or secondary device. I think people used to store all their data on floppy disks and cds so you could make copies.
- Controls: positive/negative/various controls are crucially important to be able to draw conclusions. Often times you will use your controls to normalize your data to.
- Data cleanup: Pre-processing prior to analysis is critical in lots of big data projects but also in almost every experiment. The key to this step is to make sure that you apply your pre-processing in a uniform manner to all samples and attempt to remain unbiased. In image processing, these steps may involve filtering images or adjusting brightness and contrast or cropping. Each experiment will be different but you will want to try to stay consistent across an experiment and have a good rationale for each step that you use. General things everyone will come across include the following:
o Outliers – outliers are just data points that lay outside a ‘normal’ range from the other data points you have collected. There are different ways to rationalize labelling a point as an outlier, but a common method is to remove points that are 1.5 times the interquartile range from either quartile 1 or quartile 3. This essentially tries to account for random noise or samples that might not be useful because there were errors. Removing outliers ties well into the next example (be aware that there are other methods for removing outliers, this is just the simplest one that I have seen to be common):
o Discarding results – outliers are specific points within a dataset that you wish to ignore. But sometimes an entire experiment looks a little wonky or doesn’t make sense. It isn’t valid to remove an experiment because it simply doesn’t make sense or goes against previous data. If you wish to remove results you have collected, you need a sure reason for an error in the experiment. This is where keeping an excellent lab notebook comes in handy. If you find that your concentration for a particular buffer was off, then you have an acceptable reason for discarding results. If you can’t find a valid reason, than you likely should not remove results and should just repeat the experiment again.
- Outputs: Your output from data analysis is what you present. Often times this will be a graph or a table or an image. Just make sure that your data outputs are labeled accurately, with units, and made in such a way that the data could likely be interpreted without a figure caption if possible. You should always include a figure caption, especially for papers, but if you generate your data outputs with the intent to make it possible to interpret without need for sentences, then you will likely generate a more intuitive data output.
- Statistics: it’s always nice when you can just use the FO test… is the change ‘f… obvious’. But other times you need to use a real statistical test. Note, this is very basic and general and you should really read a textbook or something. But I think this is a good general framework of the most commonly used statistics imo.
o Just get Graphpad Prism (if possible)
o Single comparison à you measure one variable in 2 groups. Are the means/medians different?
§ T-tests à use these tests when your data is sampled from a gaussian distribution (normal distribution àthere are statistical tests to determine if your data is normally distributed)
§ Mann-Whitney or Kolmogorov-Smirnov à use these tests if the data is not normally distributed (also called non-parametric)
· Mann-whitney is good for detecting shifts in the median of your 2 groups
· Kolmogorov-smirnov is good for detecting differences in the shape of you 2 groups’ distributions
o More groups à measure one variable in 3 or more groups and want to know if the means are different
§ One way ANOVA & Tukey’s
· Anova assumes a normal/gaussian/parametric distribution
· The anova only tells you if the means are different, it doesn’t specify which particular means are different from one another. Thus use the Tukey HSD to find out which means are different from which means.
§ Non-parametric Kruskal Wallis & Dunn’s post hoc
· Used for non-parametric (normal or gaussian are pretty much synonyms in my mind) comparison of 3 or more groups.
· Dunn’s post hoc is the non-parametric version of the tukey’s hsd. It will tell you which particular means are different from eachother.
§ Two-way ANOVA & Tukey’s
· If you have 2 factors. The classic example from graphpad is that you are testing 3 drugs and their effects on men vs women. Drug treatment is one factor and sex is the other.
o Multiple testing correction
§ Bonferroni – take the p-value threshold you normally use (.05) and divide it by the number of comparisons you will be making. This is your new cutoff. For example: 4 comparisons à 0.05/4 = .0125. Now your significance threshold is 0.0125.
§ Benjamini Hochberg - https://www.statisticshowto.com/benjamini-hochberg-procedure/
o Normality tests
§ D’Agostino Pearson omnibus normality test
· Tells you whether your data is normal/gaussian/parametric distribution and can help inform your decision on whether to run a parametric or nonparametric test.
Interpretation: interpreting your results can be tricky and very specific to your specific experiments. But these are a few considerations to make when speaking about your results
- Basic interpretation (descriptive)
o First it can be very helpful to describe exactly what you have done and what the output is. For example, if you ran a qpcr, then you can describe the samples that were used and how they relate to one another, and then discuss the levels of expression that have been quantified.
o Here you don’t want to make any assumptions or expand on the result. Just describe what the experiment was. specifically, do not discuss how this example indicates or suggests a greater finding. For example, if you run an experiment where you watch cells move on a 2d surface, describe it as cell migration on a 2d surface; but do not go further to say this suggests or supports a greater cell process, such as metastasis.
- Alternative hypotheses
o Be aware of other explanations for your data. Your explanation may fit, but that doesn’t necessarily mean it is the only explanation for your data. Try to be open minded and think of other possibilities.
- Proxy assay
o If you use an assay that is reductionist from another phenomena you are studying, be careful in how far you push your conclusions. For example, a simple in vitro assay is typically not sufficient to describe a complex in vivo behavior. It may be correlated and reflect an important component of the larger phenomena, but you need to be aware of the limitations of your actual study.
- Statistics
o Be careful how you describe significance. I don’t fully understand some statistics and it can be an easy place for attack by reviewers. Also it is important that you understand the significance of your own data and explicitly what it means by significance.
- Experimental weaknesses
o No experiment is perfectly a reflection of nature. This is why scientific papers include many experiments. So when interpreting your result, be aware of physical limitations in your system as well as imperfections. This is where you need to compare the actual phenomena you are interested in vs the system you are currently using.