Designing a Study
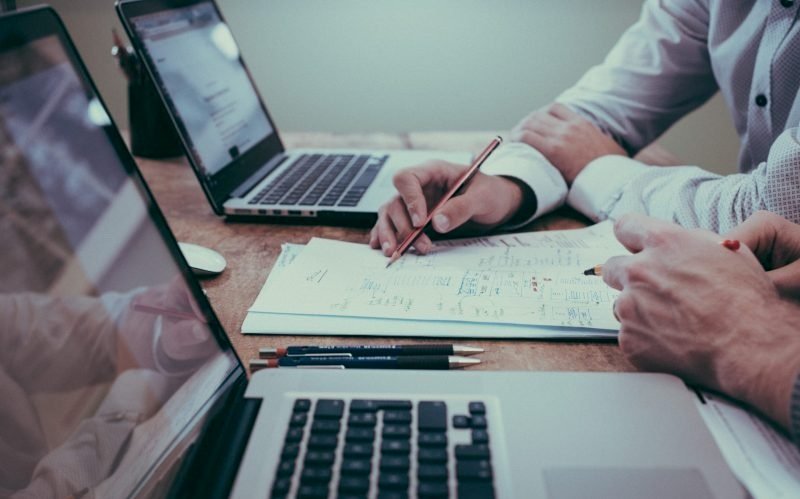
Begin with an end goal
Before jumping into the deep end of running experiments and collecting data, a good research study begins with the end goal in mind. You must have a clear idea of what you are aiming to solve or prove. What are you trying to investigate?
Requirement: Conduct a thorough background search on the topic of interest
What to look for when formulating a research idea: To get to your final goal of identifying a research question you must first understand what’s already been down in the field. Ask yourself, is your idea adding new information to the field? (i.e., improving current methods or creating new ones, new scientific findings or designs/products etc.) Do you have a clear goal/hypothesis?
Resource: Vanderbilt library has created a guide that may be useful when trying to develop a new research topic.
Formulating your research question
A research question or hypothesis needs to be clear, concise (not too broad) and have direction. Vanderbilt has provided a checklist to help you generate your research question:
Research Question Checklist:
□ Is my RQ something that I am curious about and that others might care about? Does it present an issue on which I can take a stand?
□ Does my RQ put a new spin on an old issue, or does it try to solve a problem?
□ Is my RQ too broad, too narrow, or OK?
□ Is my RQ researchable… o …within the time frame of the assignment? o …given the resources available at my location? Is my RQ measurable? What type of information do I need? Can I find actual data to support or contradict a position?
□ What sources will have the type of information that I need to answer my RQ (journals, books, internet resources, government documents, interviews with people)?
Choosing the right experimental approach
In order to answer your hypothesis or meet your goal, does your research method require a qualitative or quantitative approach or both? Choosing the right approach will help guide your experimental design.
Source: https://www.scribbr.com/research-process/research-design/
Identifying variables, controls, and sample size
VARIABLES
A variable is an important attribute that you want to study to answer your hypothesis. These can be dependent or independent depending on its cause (independent) or effect (dependent) status. Some other factors are extraneous and can lead to inaccurate conclusions if not considered during the experimental design. For example, age, gender, race, height, location, diet, habits and cultural practices etc. These are considered “confounding” and also need to be “controlled” in your experimental design if present.
SAMPLE SIZE
In order to test the validity of your study, designing experiments with “appropriate” sample size and controls are important.
QUALITATIVE APPROACH: determining the sample size for a qualitative study such as case studies involving interviews can be a bit challenging and subjective. However, here are some tips that can help:
1.
Example of confounding variables: Patients on drug A show faster recovery time compared to drug B. Does this mean that drug A is better? Or did the patient’s diet, age, or BMI also play a role in recovery time?
Quality over quantity: participants recruited should match the control or variable groups relevant to the study
2. Choose the most appropriate type of study (i.e., in-depth interview, focus groups, case studies, ethnographic etc.)
3. Consider the Principle of Saturation: “A sample size should be large enough to sufficiently describe the phenomenon of interest, and address the research question at hand. But at the same time, a large sample size risks having repetitive data”
4. See what others have published in terms of sample size in your relating field of work
QUANTITATIVE APPROACH: determining the sample size for a quantitative study is usually based on a statistical approach that considers factors such as:
1. significance level (alpha value): the probability that the difference in your data is due to randomness/chance alone. This is typically set to 0.05, meaning that there is a 95% probability that the differences seen between the control and experimental groups are scientifically and statistically significant.
2. Power: This is typically set to 80% or higher, meaning that at least 80% of the time your experiment will observe a difference between your control and experimental group if that difference exists
3. Mean differences: the average difference between the control and experimental group
Note that is method requires preliminary data before a sample size estimation can be obtained.
Experimental Design
Now that you have a clear understanding of your project goal, have formulated a question you want to explore and have identified your variables of interest, it’s time to draft an experimental procedure. This might look 100 different ways to 100 different people, even within the same field, but preparation is the key! So, here are general tips:
1. Write out your protocol before starting the actual experiment (this protocol is fluid but having a skeleton is still a great practice)
a. You can start from scratch or use previously developed methods but ensure you understand it
2. Consider your variables: what are you changing? What are you holding constant?
a. Do not change too many variables at once-this will save you a major headache when data interpreting
3. Identify all resources needed to conduct the experiment (i.e., chemicals, core facilities, equipment, personnel, etc.)
4. Use “gold-standard” methodology to validate your findings, where applicable
5. Prepare a timeline: do not try to do too much in one day you will likely make a mistake and have to start all over
a. This timeline should include instrument time especially when sharing equipment with others
DURING YOUR EXPERIMENT: RECORD EVERYTHING, DELETE NOTHING, BACKUP AND SAVE ALL FILES
Data analysis: Biostatistical tools
To determine the significance of your data, the table and resources below can help guide you in selecting the statistical tool to use based on your study groups/variables, sample size and descriptive outcomes (differences or associations).
Resources:
1. Parametric vs non-parametric test for comparing two or more groups: https://www.healthknowledge.org.uk/public-health-textbook/research-methods/1b-statistical-methods/parametric-nonparametric-tests
2. Vanderbilt Department of Biostatistics Wiki: https://biostat.app.vumc.org/wiki/Main/WebHome
a. They also have walk-in clinics to help you with your data analysis
Additional Resources:
https://samples.jbpub.com/9781284101539/9781284101539_CH06_Drummond.pdf
YOUR RESULTS MAY NOT ALWAYS BE WHAT YOU EXPECTED AND THAT IS OKAY